It is a common practice in data science to split our data up into three separate datasets in order to perform machine learning (ML) on it. The majority of the data will be used to Train our ML models and then a portion of the remaining data will be used to Validate those models. The best of these models will then be applied to our Training data to evaluate its performance.
Graphical ML tools such as Azure Machine Learning often provide easily configurable drag-and-drop tools to split our data in this manner, but what happens if we are working on a custom solution, perhaps using something like SQL Server’s In-Database Machine Learning? In this blog post we’ll look at a couple of different T-SQL solutions to quickly split our data randomly into these datasets.
A common approach is to use 60-80% of our data for the Training set and to divide the remainder evenly between Validation and Testing. For our purposes we’ll split the difference and dedicate 70% of our data to training, leaving 15% to Validation and 15% to Testing.
For these examples, we’ll be looking at patient appointment data from our sample data warehouse. Firstly we want to maintain a record of which rows of data were in which dataset for the purposes of reproducibility and tracking data lineage, so we’ll add a column to our table to hold that information.
ALTER TABLE fact.Appointment ADD Dataset VARCHAR(10) NULL;
Next we’ll update that column randomly to one of Training, Validation, or Testing based on the percentages we specified above and run a quick GROUP BY query to look at the results.
-- Method #1 - Using the RAND() function -- Definitely not the results we want! DECLARE @TrainingPercentage INT = 70, @ValidationPercentage INT = 15, @TestingPercentage INT = 15; UPDATE fact.Appointment SET Dataset = CASE WHEN 100 * RAND() < @TrainingPercentage THEN 'Training' WHEN 100 * RAND() < (@TrainingPercentage + @ValidationPercentage) THEN 'Validation' ELSE 'Testing' END; SELECT Dataset, NumberOfRows = COUNT(*) FROM fact.Appointment GROUP BY Dataset ORDER BY Dataset;
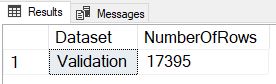
We see right away that this method failed horribly as all of the data was placed into the same dataset. This holds true no matter how many times we execute the code, and it happens because the RAND() function is only evaluated once for the whole query, and not individually for each row. To correct this we’ll instead use a method that Jeff Moden taught me at a SQL Saturday in Detroit several years ago – generating a NEWID() for each row, using the CHECKSUM() function to turn it into a random number, and then the % (modulus) function to turn it into a number between 0 and 99 inclusive.
-- Method #2 - Using the NEWID() function -- Getting closer, but still not quite there DECLARE @TrainingPercentage INT = 70, @ValidationPercentage INT = 15, @TestingPercentage INT = 15; UPDATE fact.Appointment SET Dataset = CASE WHEN ABS(CHECKSUM(NEWID())) % 100 < @TrainingPercentage THEN 'Training' WHEN ABS(CHECKSUM(NEWID())) % 100 < (@TrainingPercentage + @ValidationPercentage) THEN 'Validation' ELSE 'Testing' END; SELECT Dataset, NumberOfRows = COUNT(*) FROM fact.Appointment GROUP BY Dataset ORDER BY Dataset;
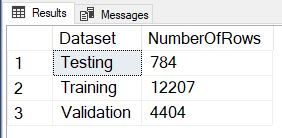
So this method failed too. Although we are getting the expected number of Training rows, the split between Validation and Testing is way off. What we find is that the NEWID() generated in the second line of the CASE statement is not the same value as in the first line, so we are getting an 85/15 split on the 30% of non-Training data instead of the expected 50/50. Let’s tweak the logic in that third line to fix the error.
-- Method #3 - Fixing the logical error -- This works, but the size of the datasets varies slightly each run DECLARE @TrainingPercentage INT = 70, @ValidationPercentage INT = 15, @TestingPercentage INT = 15; UPDATE fact.Appointment SET Dataset = CASE WHEN ABS(CHECKSUM(NEWID())) % 100 < @TrainingPercentage THEN 'Training' WHEN ABS(CHECKSUM(NEWID())) % 100 < (@ValidationPercentage * 100 / (@ValidationPercentage + @TestingPercentage)) THEN 'Validation' ELSE 'Testing' END; SELECT Dataset, NumberOfRows = COUNT(*) FROM fact.Appointment GROUP BY Dataset ORDER BY Dataset;
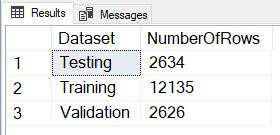
And success! The only thing we may notice is that if we execute this code multiple times, we get a slightly different number of rows in each dataset.
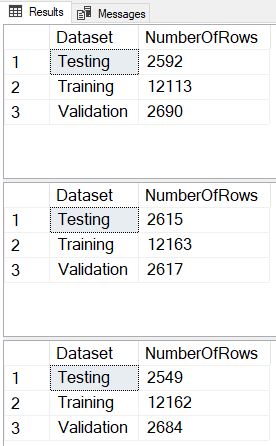
What if we have a requirement to have exactly the desired percentage of rows in each dataset, and to get that same exact percentage every time we execute? In this situation, we are going to generate a temp table that distributes the exact number of each dataset value randomly across all the rows, and then use that temp table to update the original table.
-- Method #4 - Consistent and exact DECLARE @RowCount INT, @TrainingPercentage INT = 70, @TrainingCount INT, @ValidationPercentage INT = 15, @ValidationCount INT, @TestingPercentage INT = 15, @TestingCount INT; SELECT @RowCount = COUNT(*) FROM fact.Appointment; SET @TrainingCount = (FLOOR(@RowCount * @TrainingPercentage / 100.00) + 0.5); SET @ValidationCount = (FLOOR(@RowCount * @ValidationPercentage / 100.00) + 0.5); SET @TestingCount = @RowCount - (@TrainingCount + @ValidationCount); SELECT TrainingCount = @TrainingCount, ValidationCount = @ValidationCount, TestingCount = @TestingCount; IF EXISTS (SELECT name FROM tempdb.sys.objects WHERE name LIKE '%Dataset%') DROP TABLE #Dataset; CREATE TABLE #Dataset ( AppointmentKey INT PRIMARY KEY, Dataset VARCHAR(10) NULL ); WITH SourceRows AS ( SELECT AppointmentKey, RowNumber = ROW_NUMBER() OVER (ORDER BY CHECKSUM(NEWID())) FROM fact.Appointment ) INSERT INTO #Dataset SELECT AppointmentKey, Dataset = CASE WHEN RowNumber <= @TrainingCount THEN 'Training' WHEN RowNumber <= (@TrainingCount + @ValidationCount) THEN 'Validation' ELSE 'Testing' END FROM SourceRows; UPDATE Appt SET Dataset = Dt.Dataset FROM fact.Appointment Appt INNER JOIN #Dataset Dt ON Appt.AppointmentKey = Dt.AppointmentKey SELECT Dataset, NumberOfRows = COUNT(*) FROM fact.Appointment GROUP BY Dataset ORDER BY Dataset; SELECT TOP 25 AppointmentKey, Dataset FROM fact.Appointment;
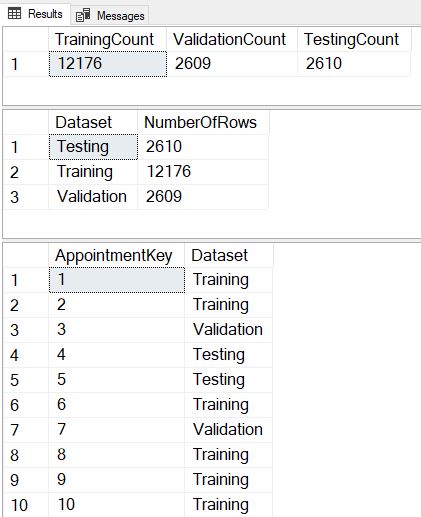
Now we see that we divide the rows in exactly the same proportions with each execution, and we’ve also included a SELECT TOP 25 so we can verify that each execution assigns different datasets to each row.
It’s possible that due to the JOIN this method might not be the best performing over extremely large datasets, so we also developed a brute-force method of splitting that performs three sequential updates that we could use instead in that specific circumstance.
-- Method #5 - The brute-force sequential method DECLARE @RowCount INT, @TrainingPercentage INT = 70, @TrainingCount INT, @ValidationPercentage INT = 15, @ValidationCount INT, @TestingPercentage INT = 15, @TestingCount INT; SELECT @RowCount = COUNT(*) FROM fact.Appointment; SET @TrainingCount = (FLOOR(@RowCount * @TrainingPercentage / 100.00) + 0.5); SET @ValidationCount = (FLOOR(@RowCount * @ValidationPercentage / 100.00) + 0.5); SET @TestingCount = @RowCount - (@TrainingCount + @ValidationCount); SELECT TrainingCount = @TrainingCount, ValidationCount = @ValidationCount, TestingCount = @TestingCount; UPDATE fact.Appointment SET Dataset = NULL; UPDATE Appt SET Dataset = 'Training' FROM ( SELECT TOP (@TrainingCount) * FROM fact.Appointment ORDER BY CHECKSUM(NEWID()) ) Appt; UPDATE Appt SET Dataset = 'Validation' FROM ( SELECT TOP (@ValidationCount) * FROM fact.Appointment WHERE Dataset IS NULL ORDER BY CHECKSUM(NEWID()) ) Appt; UPDATE fact.Appointment SET Dataset = 'Testing' WHERE Dataset IS NULL; SELECT Dataset, NumberOfRows = COUNT(*) FROM fact.Appointment GROUP BY Dataset ORDER BY Dataset; SELECT TOP 25 AppointmentKey, Dataset FROM fact.Appointment;
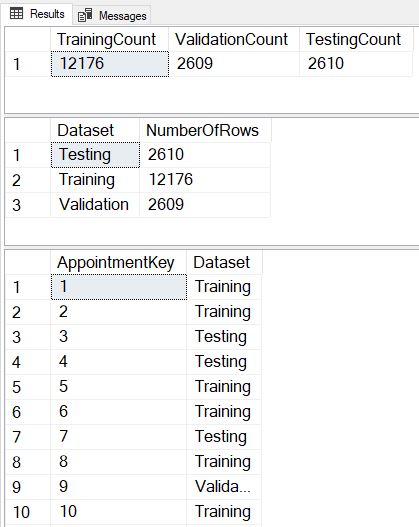
Hopefully you’ve enjoyed this look at randomly splitting our data in T-SQL. Please let me know in the comments what other methods you’ve used to tackle this problem!